14.4 非线性规划
开源的非线性优化求解器,推荐使用 nloptr,它支持全局优化,同时推荐 ROI,它有统一的接口函数。
14.4.1 一元非线性优化
下面考虑一个稍微复杂的一元函数优化问题,求复合函数的极值
\[ g(x) = \int_{0}^{x} -\sqrt{t}\exp(-t^2) \mathrm{dt}, \quad f(y) = \int_{0}^{y} g(s) \exp(-s) \mathrm{ds} \]
<- function(x) {
g integrate(function(t) {
-sqrt(t) * exp(-t^2)
lower = 0, upper = x)$value
},
}
<- function(y) {
f integrate(function(s) {
Vectorize(g, "x")(s) * exp(-s)
lower = 0, upper = y)$value
},
}
optimize(f, interval = c(10, 100), maximum = FALSE)
## $minimum
## [1] 66.84459
##
## $objective
## [1] -0.3201572
计算积分的时候,输入了一系列 s 值,参数是向量,而函数 g 只支持输入参数是单个值,g(c(1,2))
会报错,因此上面对函数 g()
用了向量化函数 Vectorize()
操作。
g(1)
## [1] -0.453392
类似地,同时计算多个目标函数 f(y)
的值,也需要Vectorize()
实现向量化操作。
Vectorize(f, "y")(c(1, 2))
## [1] -0.1103310 -0.2373865
14.4.2 多元非线性无约束优化
下面这些用来测试优化算法的函数来自维基百科
14.4.2.1 Himmelblau 函数
Himmelblau 函数是一个多摸函数,常用于比较优化算法的优劣。
\[f(x_1,x_2) = (x_1^2 + x_2 -11)^2 + (x_1 + x_2^2 -7)^2\] 它在四个位置取得一样的极小值,分别是 \(f(-3.7793, -3.2832) = 0\),\(f(-2.8051, 3.1313) = 0\),\(f(3, 2) = 0\),\(f(3.5844, -1.8481) = 0\)。函数图像见图 14.3。
# 目标函数
<- function(x) {
fn 1]^2 + x[2] - 11)^2 + (x[1] + x[2]^2 - 7)^2
(x[
}
<- expand.grid(
df x = seq(-5, 5, length = 101),
y = seq(-5, 5, length = 101)
)
$fnxy = apply(df, 1, fn)
df
library(lattice)
# 减少三维图形的边空
lattice.options(
layout.widths = list(
left.padding = list(x = -.6, units = "inches"),
right.padding = list(x = -1.0, units = "inches")
),layout.heights = list(
bottom.padding = list(x = -.8, units = "inches"),
top.padding = list(x = -1.0, units = "inches")
)
)
wireframe(
data = df, fnxy ~ x * y,
shade = TRUE, drape = FALSE,
xlab = expression(x[1]),
ylab = expression(x[2]),
zlab = list(expression(
italic(f) ~ group("(", list(x[1], x[2]), ")")
rot = 90),
), shade.colors.palette = custom_palette,
scales = list(arrows = FALSE, col = "black"),
par.settings = list(axis.line = list(col = "transparent")),
screen = list(z = -240, x = -70, y = 0)
)
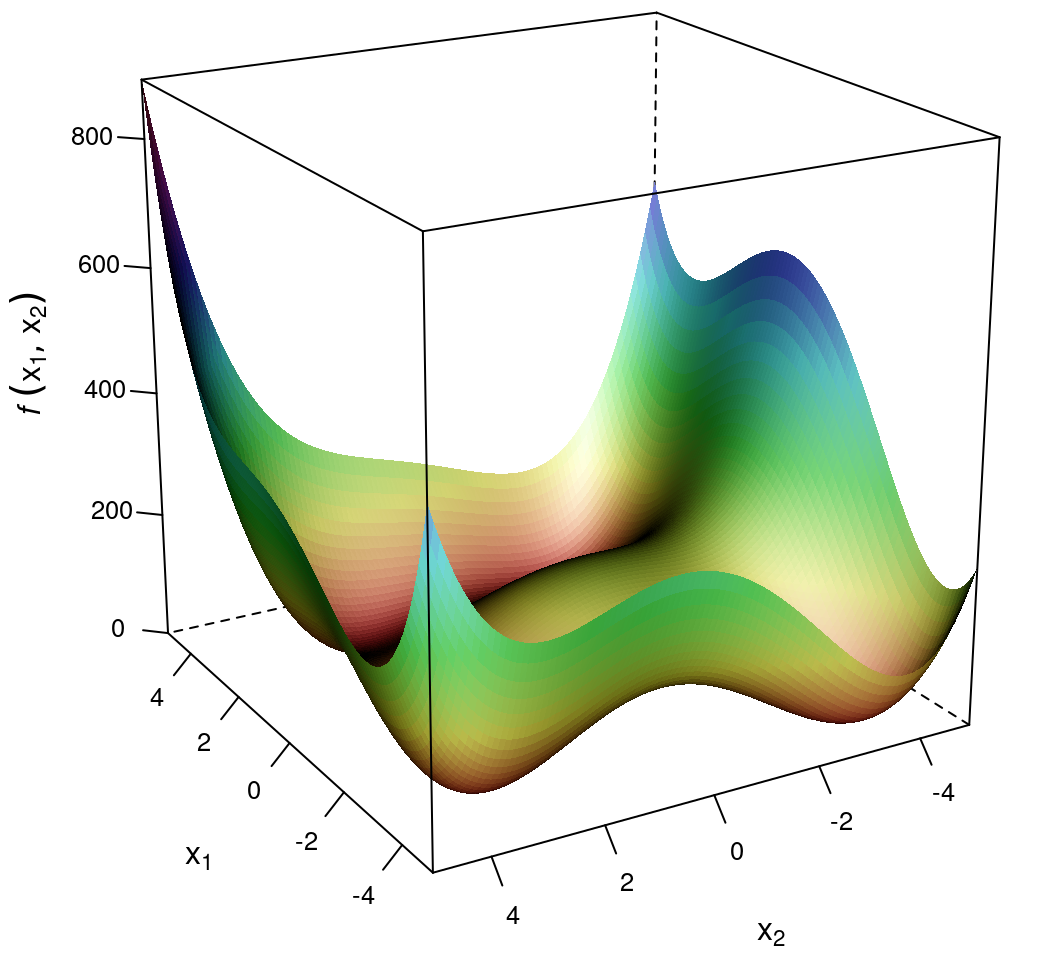
图 14.3: Himmelblau 函数图像
# 梯度函数
<- function(x) {
gr ::grad(fn, c(x[1], x[2]))
numDeriv
}optim(par = c(-1.2, 1), fn = fn, gr = gr, method = "BFGS")
## $par
## [1] -2.805118 3.131313
##
## $value
## [1] 2.069971e-27
##
## $counts
## function gradient
## 42 15
##
## $convergence
## [1] 0
##
## $message
## NULL
14.4.2.2 Peaks 函数
测试函数
\[ \begin{aligned} f(x,y) = &~ 3(1-x)\exp\{-x^2 - (y+1)^2\} \\ &- 10(\frac{x}{5} - x^3 - y^5)\exp\{-x^2-y^2\} \\ &- \frac{1}{3}\exp\{-(x+1)^2-y^2\} \end{aligned} \]
<- expression(3*(1-x)*exp^(-x^2 - (y+1)^2) - 10*(x/5 - x^3 - y^5)*exp^(-x^2-y^2) -1/3*exp^(-(x+1)^2-y^2)) peaks
D(peaks, "x")
## -(3 * (1 - x) * (exp^(-x^2 - (y + 1)^2) * (log(exp) * (2 * x))) +
## 3 * exp^(-x^2 - (y + 1)^2) + (10 * (1/5 - 3 * x^2) * exp^(-x^2 -
## y^2) - 10 * (x/5 - x^3 - y^5) * (exp^(-x^2 - y^2) * (log(exp) *
## (2 * x)))) - 1/3 * (exp^(-(x + 1)^2 - y^2) * (log(exp) *
## (2 * (x + 1)))))
D(peaks, "y")
## -(3 * (1 - x) * (exp^(-x^2 - (y + 1)^2) * (log(exp) * (2 * (y +
## 1)))) - (10 * (x/5 - x^3 - y^5) * (exp^(-x^2 - y^2) * (log(exp) *
## (2 * y))) + 10 * (5 * y^4) * exp^(-x^2 - y^2)) - 1/3 * (exp^(-(x +
## 1)^2 - y^2) * (log(exp) * (2 * y))))
library(Deriv)
Simplify(D(peaks, "x"))
## -(10 * ((0.2 - 3 * x^2)/exp^(x^2 + y^2)) + 3/exp^((1 + y)^2 +
## x^2) + log(exp) * (x * (6 * ((1 - x)/exp^((1 + y)^2 + x^2)) -
## 20 * ((x * (0.2 - x^2) - y^5)/exp^(x^2 + y^2))) - 0.666666666666667 *
## ((1 + x)/exp^((1 + x)^2 + y^2))))
Simplify(D(peaks, "y"))
## -((6 * ((1 - x) * (1 + y)/exp^((1 + y)^2 + x^2)) - 0.666666666666667 *
## (y/exp^((1 + x)^2 + y^2))) * log(exp) - y * (20 * (log(exp) *
## (x * (0.2 - x^2) - y^5)/exp^(x^2 + y^2)) + 50 * (y^3/exp^(x^2 +
## y^2))))
<- function(x) {
fn 3 * (1 - x[1])^2 * exp(-x[1]^2 - (x[2] + 1)^2) -
10 * (x[1] / 5 - x[1]^3 - x[2]^5) * exp(-x[1]^2 - x[2]^2) -
1 / 3 * exp(-(x[1] + 1)^2 - x[2]^2)
}# 梯度函数
<- function(x) {
gr ::grad(fn, c(x[1], x[2]))
numDeriv
}
optim(par = c(-1.2, 1), fn = fn, gr = gr, method = "BFGS")
## $par
## [1] -1.3473958 0.2045192
##
## $value
## [1] -3.049849
##
## $counts
## function gradient
## 28 10
##
## $convergence
## [1] 0
##
## $message
## NULL
在 \((-1.3473958, 0.2045192)\) 处取得极小值
<- expand.grid(
df x = seq(-3, 3, length = 51),
y = seq(-3, 3, length = 51)
)
$fnxy <- apply(df, 1, fn)
df
wireframe(
data = df, fnxy ~ x * y,
shade = TRUE, drape = FALSE,
xlab = expression(x[1]),
ylab = expression(x[2]),
zlab = list(expression(
italic(f) ~ group("(", list(x[1], x[2]), ")")
rot = 90), aspect = c(1, 0.75),
), shade.colors.palette = custom_palette,
scales = list(arrows = FALSE, col = "black"),
par.settings = list(
axis.line = list(col = "transparent")
),screen = list(z = 45, x = -70, y = 0)
)
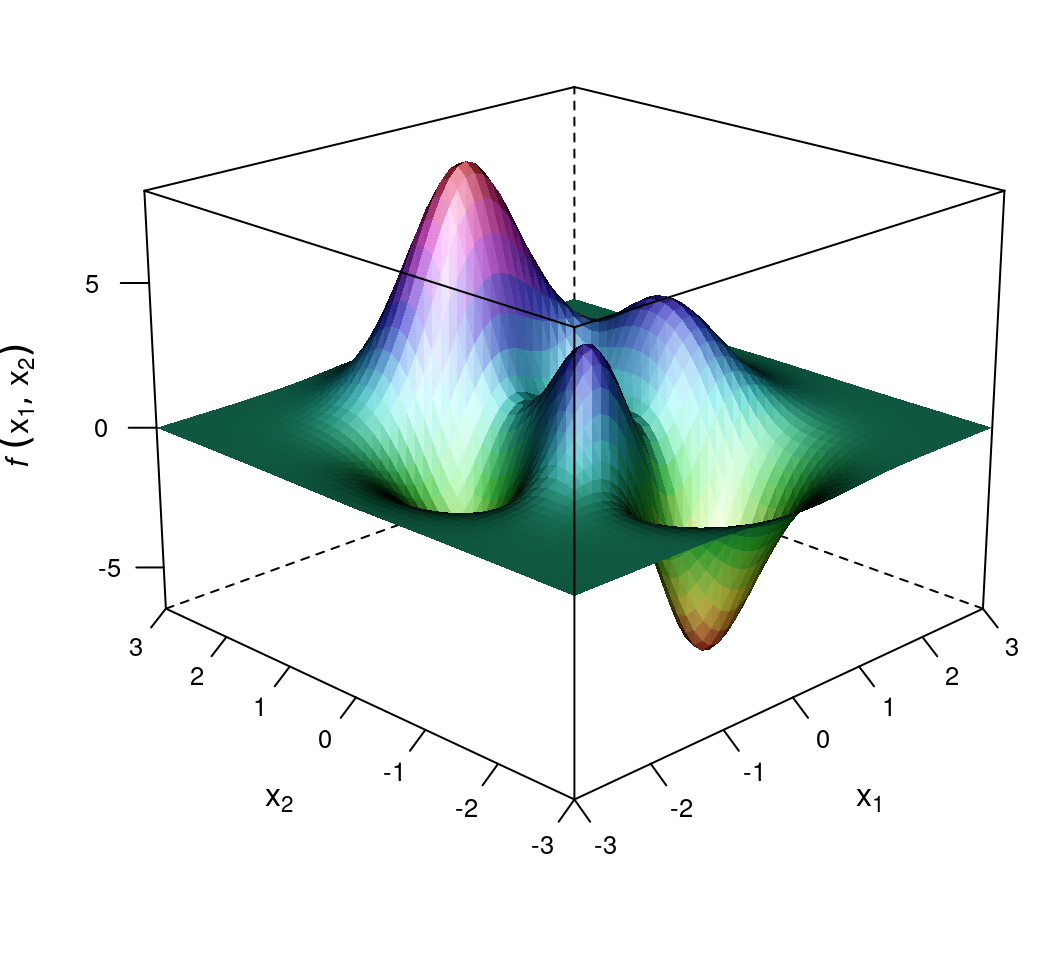
图 14.4: Peaks 多峰图像
函数来自 Octave 内置的 peaks()
函数,它有很多的局部极大值和极小值,可在 Octave Online 上输入命令 help peaks
查看其帮助文档。
14.4.2.3 Rosenbrock 函数
香蕉函数定义如下:
\[f(x_1,x_2) = 100 (x_2 -x_1^2)^2 + (1 - x_1)^2\]
<- function(x) {
fn 100 * (x[2] - x[1]^2)^2 + (1 - x[1])^2)
(
}
<- expand.grid(
df x = seq(-2.5, 2.5, length = 101),
y = seq(-2.5, 2.5, length = 101)
)$fnxy = apply(df, 1, fn)
df
wireframe(
data = df, fnxy ~ x * y,
shade = TRUE, drape = FALSE,
xlab = expression(x[1]),
ylab = expression(x[2]),
zlab = list(expression(italic(f) ~ group("(", list(x[1], x[2]), ")")), rot = 90),
scales = list(arrows = FALSE, col = "black"),
par.settings = list(axis.line = list(col = "transparent")),
screen = list(z = 120, x = -70, y = 0)
)
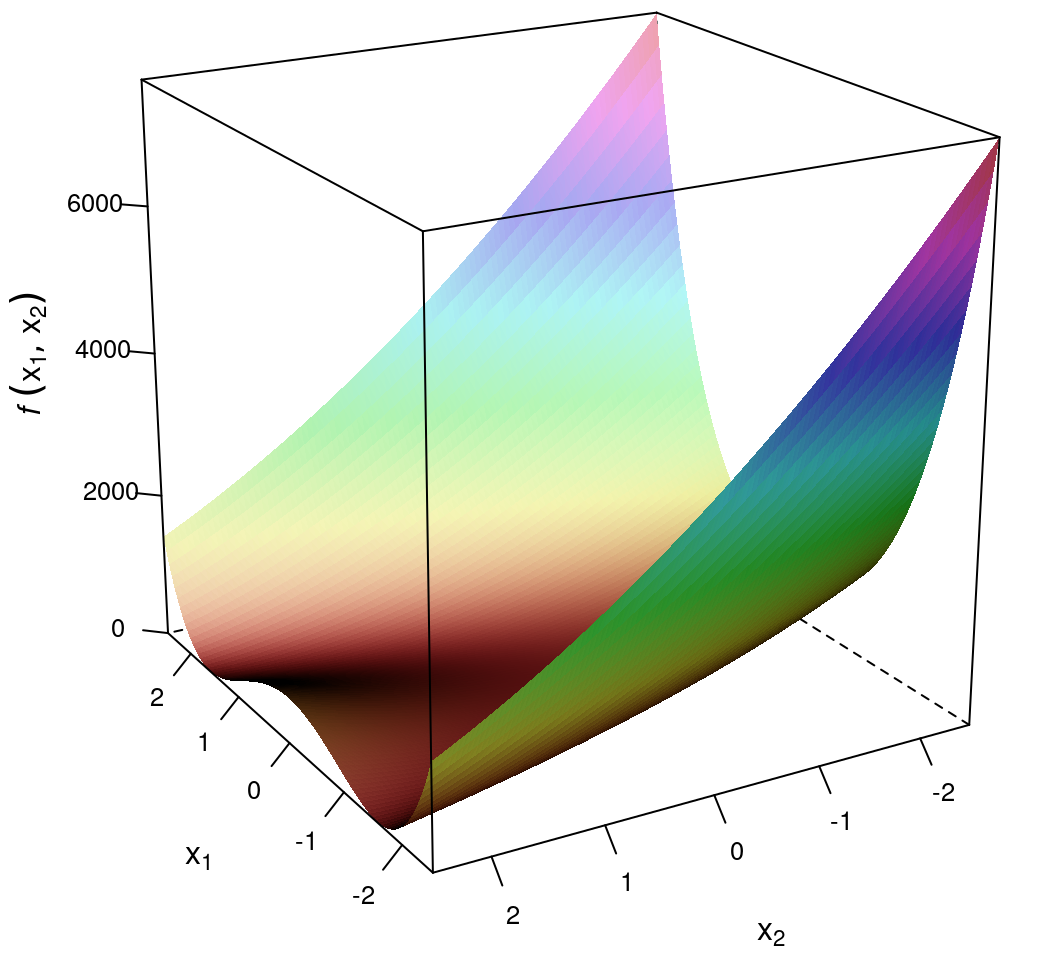
图 14.5: 香蕉函数图像
<- raster::rasterFromXYZ(df, crs = CRS("+proj=longlat +datum=WGS84"))
r ::vectorplot(r, par.settings = RdBuTheme()) rasterVis
# 梯度函数
<- function(x) {
gr ::grad(fn, c(x[1], x[2]))
numDeriv
}optim(par = c(-1.2, 1), fn = fn, gr = gr, method = "BFGS")
## $par
## [1] 1 1
##
## $value
## [1] 9.595012e-18
##
## $counts
## function gradient
## 110 43
##
## $convergence
## [1] 0
##
## $message
## NULL
<- OP(
op objective = F_objective(fn, n = 2L, G = gr),
bounds = V_bound(ld = -3, ud = 3, nobj = 2L)
)<- ROI_solve(op, solver = "nloptr.lbfgs", start = c(-1.2, 1))
nlp $objval nlp
## [1] 1.364878e-17
$solution nlp
## [1] 1 1
14.4.2.4 Ackley 函数
Ackley 函数是一个非凸函数,有大量局部极小值点,获取全局极小值点是一个比较有挑战的事。它的 \(n\) 维形式如下: \[f(\mathbf{x}) = - a \mathrm{e}^{-b\sqrt{\frac{1}{n}\sum_{i=1}^{n}x_{i}^{2}}} - \mathrm{e}^{\frac{1}{n}\sum_{i=1}^{n}\cos(cx_i)} + a + \mathrm{e}\] 其中,\(a = 20, b = 0.2, c = 2\pi\),对 \(\forall i = 1,2,\cdots, n\),\(x_i \in [-10, 10]\),\(f(\mathbf{x})\) 在 \(\mathbf{x}^{\star} = (0,0,\cdot,0)\) 取得全局最小值 \(f(\mathbf{x}^{\star}) = 0\),二维图像如图 14.6。
<- function(x, a = 20, b = 0.2, c = 2 * pi) {
fn <- mean(x^2)
mean1 <- mean(cos(c * x))
mean2 -a * exp(-b * sqrt(mean1)) - exp(mean2) + a + exp(1)
}
<- expand.grid(
df x = seq(-10, 10, length.out = 201),
y = seq(-10, 10, length.out = 201)
)
$fnxy = apply(df, 1, fn)
df
wireframe(
data = df, fnxy ~ x * y,
shade = TRUE, drape = FALSE,
xlab = expression(x[1]),
ylab = expression(x[2]),
zlab = list(expression(italic(f) ~ group("(", list(x[1], x[2]), ")")), rot = 90),
scales = list(arrows = FALSE, col = "black"),
par.settings = list(axis.line = list(col = "transparent")),
screen = list(z = 120, x = -70, y = 0)
)
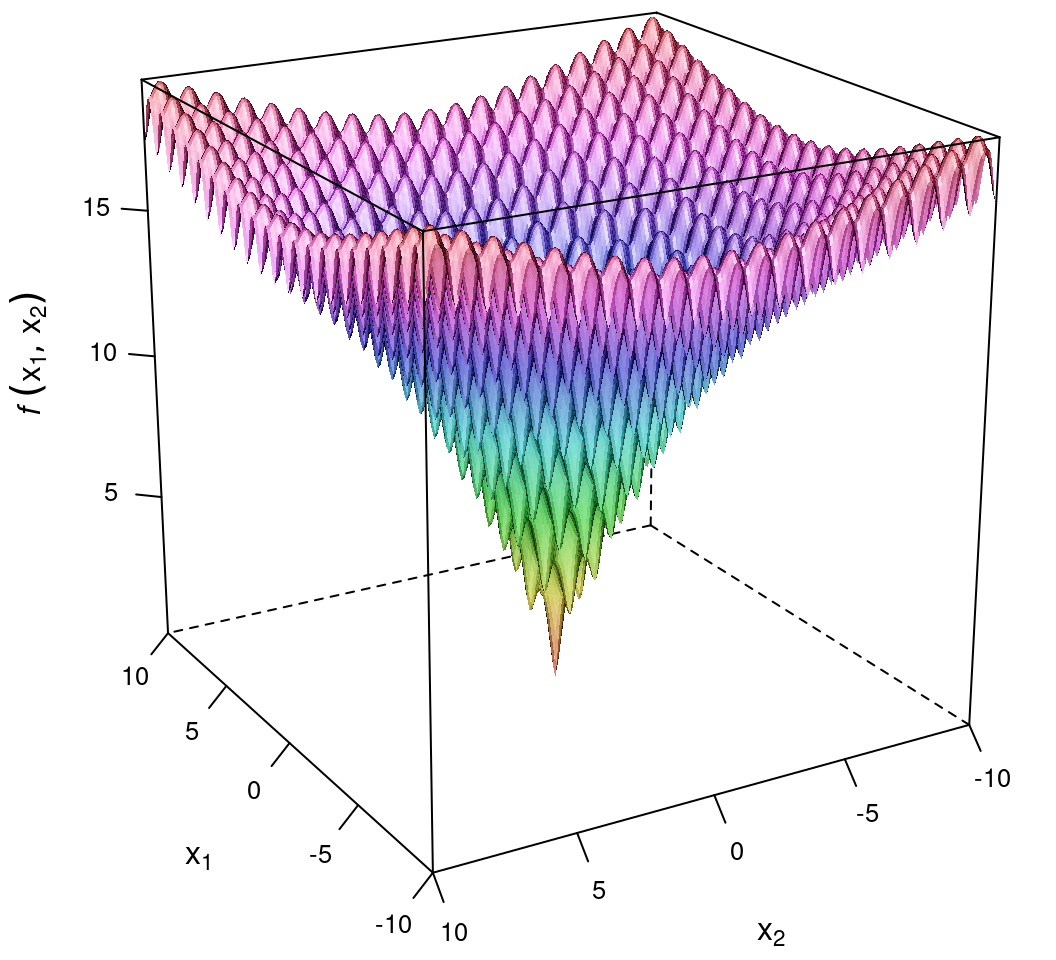
图 14.6: 二维 Ackley 函数图像
以 10 维的 Ackley 函数为例,先试一下普通的局部优化算法 — Nelder–Mead 算法,选择初值 \((2,2,\cdots,2)\) ,看下效果,再与全局优化算法比较。
<- OP(
op objective = F_objective(fn, n = 10L),
bounds = V_bound(ld = -10, ud = 10, nobj = 10L)
)
<- ROI_solve(op, solver = "nloptr.neldermead", start = rep(2, 10))
nlp $solution nlp
## [1] 2 2 2 2 2 2 2 2 2 2
$objval nlp
## [1] 6.593599
可以说完全没有优化效果,已经陷入局部极小值。根据nloptr 全局优化算法的介绍,这里采用 directL 算法,因为是全局优化,不用选择初值。
# 调全局优化器
<- ROI_solve(op, solver = "nloptr.directL")
nlp $solution nlp
## [1] 0 0 0 0 0 0 0 0 0 0
$objval nlp
## [1] 4.440892e-16
fn(x = c(2, 2))
## [1] 6.593599
fn(x = rep(2, 10))
## [1] 6.593599
14.4.2.5 Schaffer 函数
\[ f(x_1,x_2) = 0.5 + \frac{\sin^2(x_1^2 - x_2^2) - 0.5}{ [1 + 0.001(x_1^2 + x_2^2)]^2} \]
在 \(\mathbf{x}^\star = (0,0)\) 处取得全局最小值 \(f(\mathbf{x}^\star) = 0\)
<- function(x) {
fn 0.5 + ((sin(x[1]^2 - x[2]^2))^2 - 0.5) / (1 + 0.001*(x[1]^2 + x[2]^2))^2
}
<- expand.grid(
df x = seq(-50, 50, length = 201),
y = seq(-50, 50, length = 201)
)$fnxy = apply(df, 1, fn)
df
wireframe(
data = df, fnxy ~ x * y,
shade = TRUE, drape = FALSE,
xlab = expression(x[1]),
ylab = expression(x[2]),
zlab = list(expression(italic(f) ~ group("(", list(x[1], x[2]), ")")), rot = 90),
scales = list(arrows = FALSE, col = "black"),
par.settings = list(axis.line = list(col = "transparent")),
screen = list(z = 120, x = -70, y = 0)
)
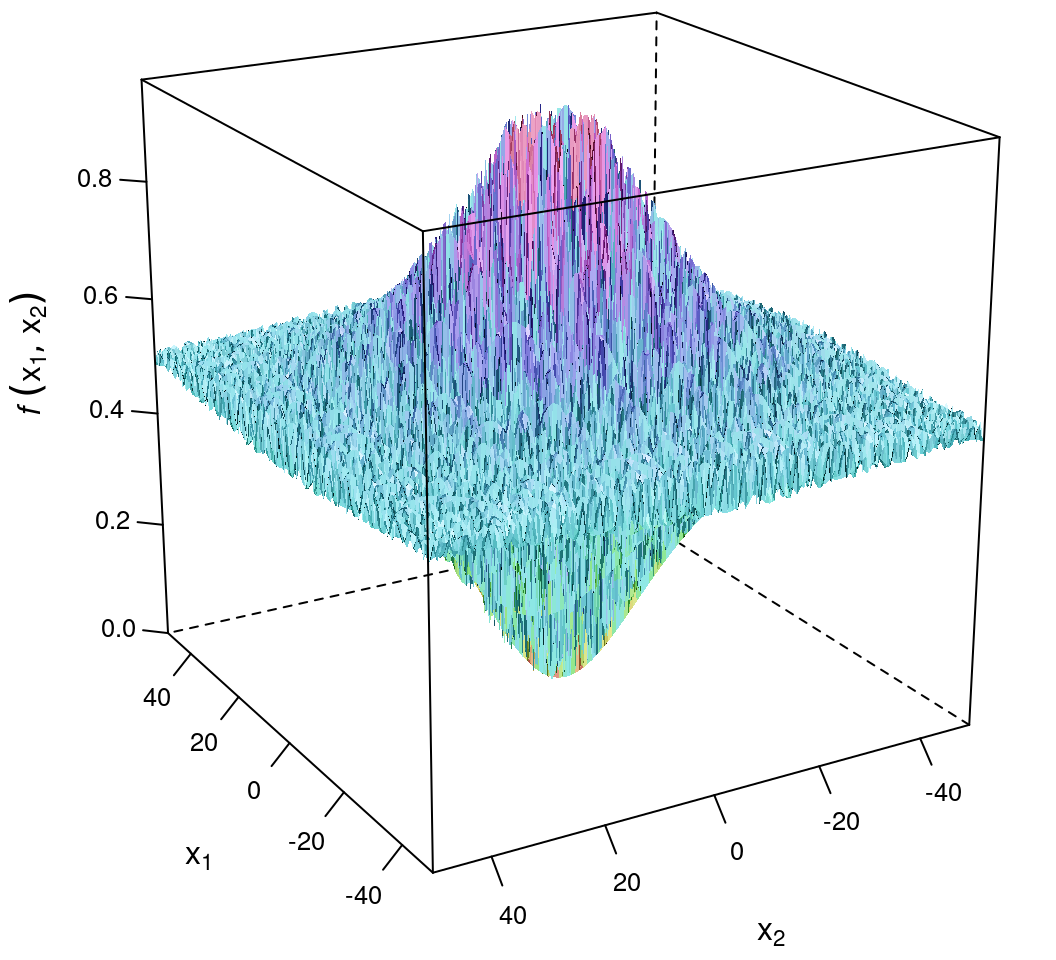
图 14.7: Schaffer 函数
<- expand.grid(
df x = seq(-2, 2, length = 101),
y = seq(-2, 2, length = 101)
)$fnxy = apply(df, 1, fn)
df
wireframe(
data = df, fnxy ~ x * y,
shade = TRUE, drape = FALSE,
xlab = expression(x[1]),
ylab = expression(x[2]),
zlab = list(expression(italic(f) ~ group("(", list(x[1], x[2]), ")")), rot = 90),
scales = list(arrows = FALSE, col = "black"),
par.settings = list(axis.line = list(col = "transparent")),
screen = list(z = 120, x = -70, y = 0)
)
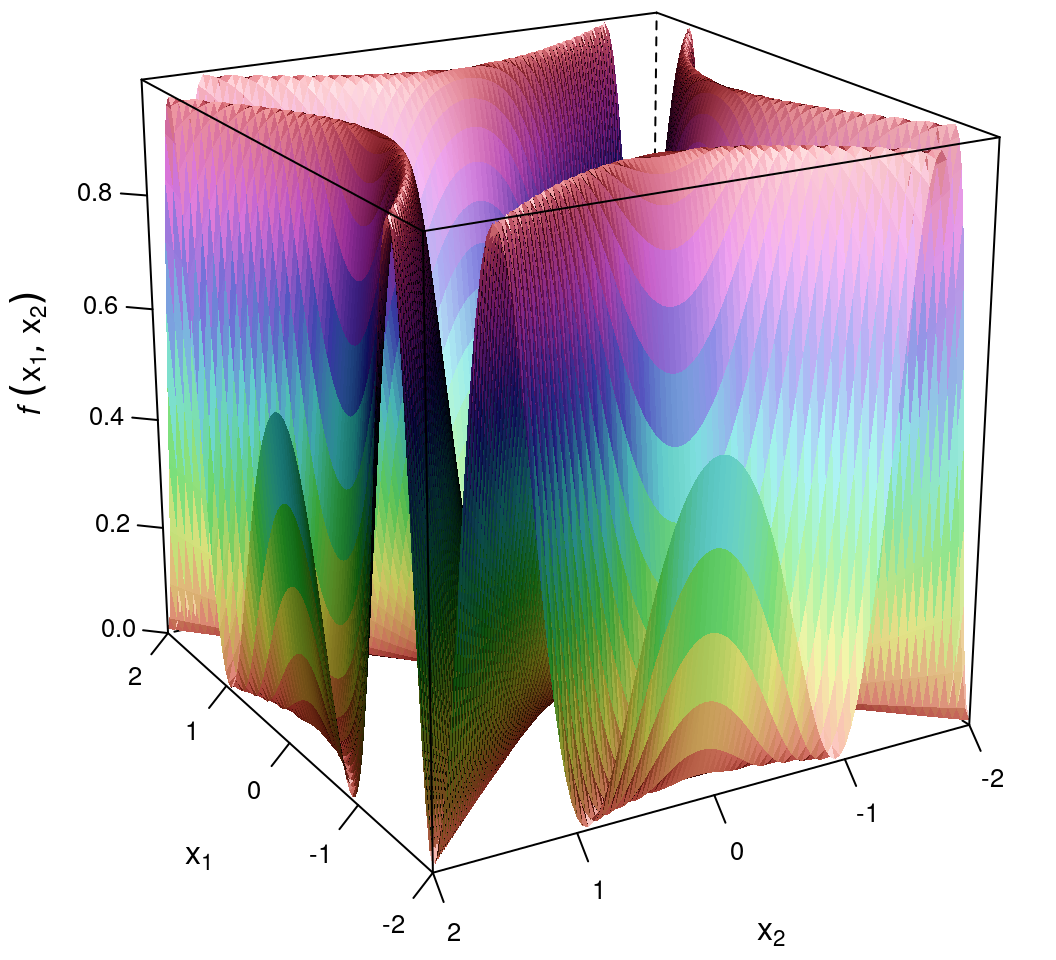
图 14.8: Schaffer 函数
14.4.2.6 Hölder 函数
Hölder 桌面函数
\[ f(x_1,x_2) = - | \sin(x_1)\cos(x_2)\exp\big(| 1 - \frac{\sqrt{x_1^2 + x_2^2}}{\pi}|\big) | \]
在 \((8.05502, 9.66459)\)、\((-8.05502, 9.66459)\)、\((8.05502, -9.66459)\)、\((-8.05502, -9.66459)\) 同时取得最小值 \(-19.2085\)。
<- function(x) {
fn -abs(sin(x[1]) * cos(x[2])) * exp(abs(1 - sqrt(x[1]^2 + x[2]^2) / pi))
}
<- expand.grid(
df x = seq(-10, 10, length = 101),
y = seq(-10, 10, length = 101)
)$fnxy = apply(df, 1, fn)
df
wireframe(
data = df, fnxy ~ x * y,
shade = TRUE, drape = FALSE,
xlab = expression(x[1]),
ylab = expression(x[2]),
zlab = list(expression(italic(f) ~ group("(", list(x[1], x[2]), ")")), rot = 90),
scales = list(arrows = FALSE, col = "black"),
par.settings = list(axis.line = list(col = "transparent")),
screen = list(z = 120, x = -60, y = 0)
)
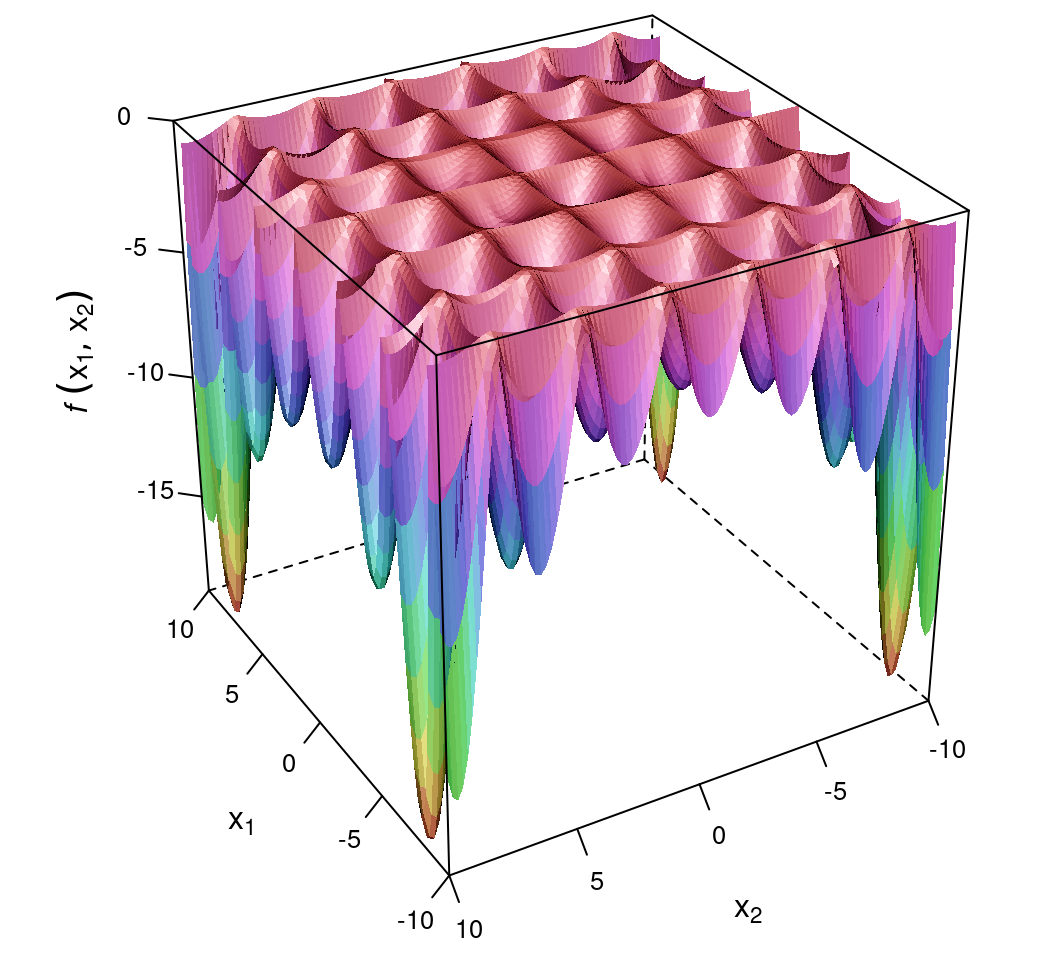
图 14.9: Hölder 函数
14.4.2.7 Trid 函数
\(n \geq 2\) 维 Trid 函数
\[ f(x) = \sum_{i=1}^{n}(x_i - 1)^2 - \sum_{i=2}^{n}x_i x_{i-1} \] \(\forall i = 1,2,\cdots, n\),\(f(x)\) 在 \(x_i = i(n+1-i)\) 处取得全局极小值 \(f(\mathbf{x}^\star)=-n(n+4)(n-1)/6\),取值区间 \(x \in [-n^2, n^2], \forall i = 1,2,\cdots,n\)
<- function(x) {
fn <- length(x)
n sum((x - 1)^2) - sum(x[-1] * x[-n])
}
<- expand.grid(
df x = seq(-4, 4, length = 101),
y = seq(-4, 4, length = 101)
)$fnxy = apply(df, 1, fn)
df
wireframe(
data = df, fnxy ~ x * y,
shade = TRUE, drape = FALSE,
xlab = expression(x[1]),
ylab = expression(x[2]),
zlab = list(expression(italic(f) ~ group("(", list(x[1], x[2]), ")")), rot = 90),
scales = list(arrows = FALSE, col = "black"),
par.settings = list(axis.line = list(col = "transparent")),
screen = list(z = -60, x = -70, y = 0)
)
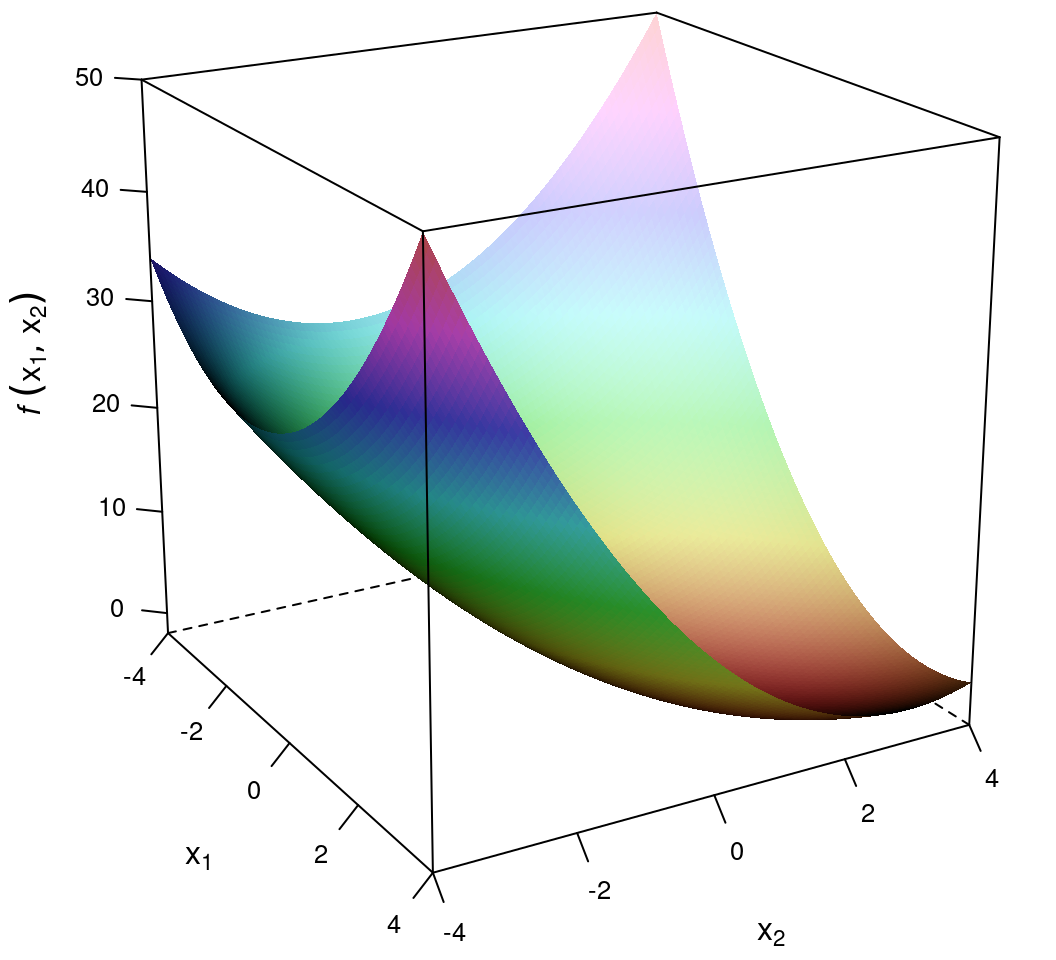
图 14.10: Trid 函数
14.4.3 多元非线性约束优化
14.4.3.1 非线性严格不等式约束
第一个例子,目标函数是非线性的,约束条件也是非线性的,非线性不等式约束不包含等号。
\[\begin{equation*} \begin{array}{l} \min_x \quad (x_1 + 3x_2 + x_3)^2 + 4(x_1 - x_2)^2 \\ s.t.\left\{ \begin{array}{l} x_1 + x_2 + x_3 = 1 \\ 6 x_2 + 4 x_3 - x_1^3 > 3 \\ x_1, x_2, x_3 > 0 \end{array} \right. \end{array} \end{equation*}\]# 目标函数
<- function(x) (x[1] + 3 * x[2] + x[3])^2 + 4 * (x[1] - x[2])^2
fn # 目标函数的梯度
<- function(x) {
gr c(
2 * (x[1] + 3 * x[2] + x[3]) + 8 * (x[1] - x[2]), # 对 x[1] 求偏导
6 * (x[1] + 3 * x[2] + x[3]) - 8 * (x[1] - x[2]), # 对 x[2] 求偏导
2 * (x[1] + 3 * x[2] + x[3]) # 对 x[3] 求偏导
)
}# 等式约束
<- function(x) {
heq 1] + x[2] + x[3] - 1
x[
}# 等式约束的雅可比矩阵
# 这里只有一个等式约束,所以雅可比矩阵行数为 1
<- function(x) {
heq.jac matrix(c(1, 1, 1), ncol = 3, byrow = TRUE)
}# 不等式约束
# 要求必须是严格不等式,不能带等号,方向是 x > 0
<- function(x) {
hin c(6 * x[2] + 4 * x[3] - x[1]^3 - 3, x[1], x[2], x[3])
}# 不等式约束的雅可比矩阵
# 其实是有 4 个不等式约束,3 个目标变量约束,雅可比矩阵行数是 4
<- function(x) {
hin.jac matrix(c(
-3 * x[1]^2, 6, 4,
1, 0, 0,
0, 1, 0,
0, 0, 1
ncol = 3, byrow = TRUE)
), }
调用 alabama 包的求解器
set.seed(12)
# 初始值
<- runif(3)
p0 # 求目标函数的极小值
<- alabama::constrOptim.nl(
ans par = p0,
# 目标函数
fn = fn,
gr = gr,
# 等式约束
heq = heq,
heq.jac = heq.jac,
# 不等式约束
hin = hin,
hin.jac = hin.jac,
# 不显示迭代过程
control.outer = list(trace = FALSE)
) ans
## $par
## [1] 7.390292e-04 4.497160e-12 9.992610e-01
##
## $value
## [1] 1.000002
##
## $counts
## function gradient
## 1230 163
##
## $convergence
## [1] 0
##
## $message
## NULL
##
## $hessian
## [,1] [,2] [,3]
## [1,] 120517098 120517087 120517091
## [2,] 120517087 120517115 120517095
## [3,] 120517091 120517095 120517091
##
## $outer.iterations
## [1] 13
##
## $lambda
## [1] 4.481599
##
## $sigma
## [1] 120517089
##
## $barrier.value
## [1] 0.003472071
##
## $K
## [1] 4.269112e-08
ans 是 constrOptim.nl()
返回的一个 list, convergence = 0 表示迭代成功收敛,value 表示目标函数在迭代终止时的取直,par 表示满足约束条件,成功收敛的情况下,目标函数的参数值,counts 表示迭代过程中目标函数及其梯度计算的次数。
# 不提供梯度函数,照样可以求解
<- alabama::constrOptim.nl(par = p0, fn = fn, heq = heq, hin = hin) ans
等式和不等式约束的雅可比矩阵必须以 matrix 数据类型存储,而不能以 vector 类型存储。要注意和后面 ROI 包的调用形式区别。
实际上,可以用 ROI 调用 alabama 求解器的方式,这种方式可以简化目标函数梯度和约束条件的表示
# 目标函数
<- function(x) (x[1] + 3 * x[2] + x[3])^2 + 4 * (x[1] - x[2])^2
fn # 目标函数的梯度
<- function(x) {
gr c(
2 * (x[1] + 3 * x[2] + x[3]) + 8 * (x[1] - x[2]),
6 * (x[1] + 3 * x[2] + x[3]) - 8 * (x[1] - x[2]),
2 * (x[1] + 3 * x[2] + x[3])
)
}<- function(x) {
heq 1] + x[2] + x[3]
x[
}<- function(x) {
heq.jac c(1, 1, 1)
}<- function(x) {
hin 6 * x[2] + 4 * x[3] - x[1]^3
}<- function(x) {
hin.jac c(-3 * x[1]^2, 6, 4)
}
通过 ROI 调用 alabama 求解器
set.seed(2020)
# 初始值
<- runif(3)
p0 # 定义目标规划
<- OP(
op objective = F_objective(F = fn, n = 3L, G = gr), # 4 个目标变量
constraints = F_constraint(
F = list(heq = heq, hin = hin),
dir = c("==", ">"),
rhs = c(1, 3),
# 等式和不等式约束的雅可比
J = list(heq.jac = heq.jac, hin.jac = hin.jac)
),bounds = V_bound(ld = 0, ud = +Inf, nobj = 3L),
maximum = FALSE # 求最小
)<- ROI_solve(op, solver = "alabama", start = p0)
nlp $solution nlp
## [1] 1.674812e-06 9.994336e-08 9.999982e-01
$objval nlp
## [1] 1
14.4.3.2 非线性混合整数约束
\[\begin{equation*} \begin{array}{l} \max_x \quad 1.5(x_1 - \sin(x_1 - x_2))^2 + 0.5x_2^2 + x_3^2 - x_1 x_2 - 2x_1 + x_2 x_3 \\ s.t.\left\{ \begin{array}{l} -20 < x_1 < 20 \\ -20 < x_2 < 20 \\ -10 < x_3 < 10 \\ x_1, x_2 \in \mathbb{R}, \quad x_3 \in \mathbb{Z} \end{array} \right. \end{array} \end{equation*}\]<- function(x) {
fn 1.5 * (x[1] - sin(x[1] - x[2]))^2 + 0.5 * x[2]^2 + x[3]^2
-x[1] * x[2] - 2 * x[1] + x[2] * x[3]
}<- function(x) {
gr c(
3 * (x[1] - sin(x[1] - x[2])) * (1 - cos(x[1] - x[2])) - x[2] - 2,
3 * (x[1] - sin(x[1] - x[2])) * cos(x[1] - x[2]) - x[2] - x[1] + x[3],
2 * x[3] + x[2]
) }
目前 ROI 还解不了
# 初始值
<- c(2.1, 5.1, 5)
p0 # 定义目标规划
<- OP(
op objective = F_objective(F = fn, n = 3L, G = gr), # 3 个目标变量
types = c("C", "C", "I"), # 目标变量的类型
bounds = V_bound(lb = c(-20, -20, -10), ub = c(20, 20, 10), nobj = 3L),
maximum = FALSE # 求最小
)<- ROI_solve(op, solver = "auto", start = p0)
nlp $solution nlp
目标函数在 \((4.49712, 9.147501, -4)\) 取得最小值 -86.72165
fn(x = c(4.49712, 9.147501, -4))
## [1] -86.72165
14.4.3.3 含复杂目标函数
下面这个目标函数比较复杂,约束条件也是非线性的
\[\begin{equation*} \begin{array}{l} \max_x \quad \frac{(\sin(2\pi x_1))^3 \sin(2\pi x_2)}{x_1^3 (x_1 + x_2)} \\ s.t.\left\{ \begin{array}{l} x_1^2 - x_2 + 1 \leq 0 \\ 1 - x_1 + (x_2 - 4)^2 \geq 0 \\ 0 \leq x_1, x_2 \leq 10 \end{array} \right. \end{array} \end{equation*}\]# 目标函数
<- function(x) (sin(2*pi*x[1]))^3 * sin(2*pi*x[2])/(x[1]^3*(x[1] + x[2]))
fn # 目标函数的梯度
<- function(x) {
gr ::grad(fn, c(x[1], x[2]))
numDeriv
}
<- function(x) {
hin c(
1]^2 - x[2] + 1,
x[1 - x[1] + (x[2] - 4)^2
)
}
<- function(x) {
hin.jac matrix(c(
2 * x[1], -1,
-1, 2 * x[2]
),ncol = 2, byrow = TRUE
)
}
# 初始值
<- c(2, 5)
p0 # 定义目标规划
<- OP(
op objective = F_objective(F = fn, n = 2L, G = gr), # 2 个目标变量
constraints = F_constraint(
F = list(hin = hin),
dir = c("<=", "<="),
rhs = c(0, 0),
# 不等式约束的雅可比
J = list(hin.jac = hin.jac)
),bounds = V_bound(ld = 0, ud = 10, nobj = 2L),
maximum = TRUE # 求最大
)<- ROI_solve(op, solver = "nloptr.isres", start = p0)
nlp $solution nlp
## [1] 1.227969 4.245379
$objval nlp
## [1] 0.09582504